Blog post
How Microsoft Advertising is revolutionizing productivity with AI
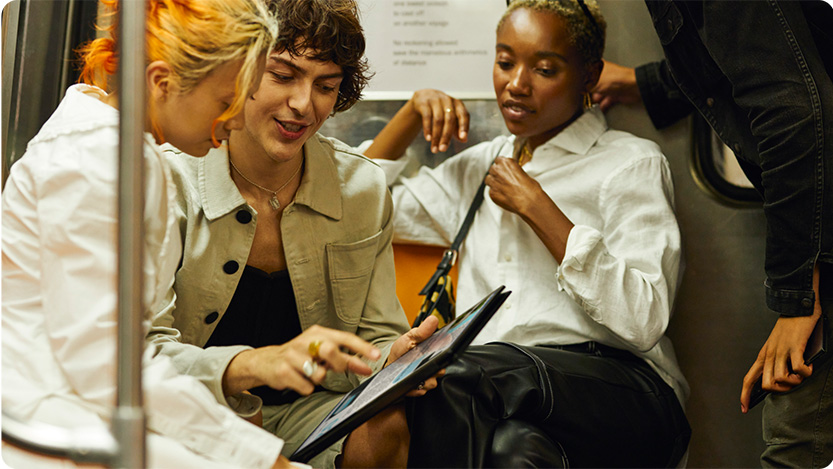
A three-part series on how generative AI can empower advertising and marketing professionals to achieve more
Welcome to a new blog series where we will share our journey of deploying generative AI solutions to boost productivity, creativity, and collaboration of our team of advertising and marketing professionals at Microsoft Advertising. We will offer tips and insights on how you can leverage Copilot for Microsoft 365, a powerful AI-enabled tool that can help you create high-quality content faster and more thoughtfully.
In this series, we will cover the following topics:
- Power user's guide to AI for advanced scenarios - in our first blog post, using the concept of personas, we will focus on how advertising and marketing professionals can skill up and train themselves to use AI effectively and confidently in their work.
- Think, share, do: Three steps to enhanced-AI collaboration - our second blog post will offer a framework demonstrating how people can collaborate better with their peers and teams using AI and Copilot for Microsoft 365.
- Rebalancing the work equation with AI - our third blog post will explore how people can save time and create new opportunities with AI and Copilot for Microsoft 365.
But before we dive into these topics, we want to give you background on how we developed and deployed a generative AI framework, which consists of four pillars: identify, build, train, and measure. These are the essential steps that we followed to launch AI technologies, like Insights Navigator and CoPlanner, that reduce our manual workflows and unlock creativity.
Identify: Think outside the box
When starting a new generative AI project, the most important step is to understand the pain points of your audience. While it is easiest to target tedious workflows, generative AI does not have to be limited to productivity improvements only. Understanding problems in your organization means thinking “outside the box,” as opposed to looking for areas where chat bots make common sense. With each capability that we've developed in-house and deployed to our employees, we’ve gone through a thoughtful process to first identify the most critical use cases based on time spent, bottom line cost reduction, or potential top line revenue growth. Then the use cases are prioritized relative to business priorities and technical feasibility. Each area of focus requires collaboration across work groups to ensure the prevailing pain point could be addressed with AI technology readily available. With thorough discovery and clear problem statements, we could then confidently move forward.
Build: Bridging teams and technology
After discovery is complete the next step is to build. There are a variety of factors to consider as part of the build, such as: data availability, privacy, compliance, security and trust of utilizing said data, the tenants of responsible AI, and ensuring there are not duplicative AI toolsets within the organization. These checks are crucial, even for an internal solution, to preserve the original intent of the data source, the conditions under which the data was collected, and appropriate transformation and processing based on guiding principles of responsible AI. As development progresses, it is important to check in with stakeholders, keeping them close to the process to ensure the output speeds up resolution of their workflow and is adequately tested for inaccurate response information, hallucinations, etc. These things come together to increase adoption, speed to market, and accelerate the realization of value from investments in generative AI.
Train: Learning path evolution
We've learned first-hand in the last year how essential training is for new technologies and capabilities. Time is short, and time to invest in learning tends to be even shorter. However, AI is only an effective tool when behaviors change to adopt it. As users move from discovery, to adoption, and finally to becoming power users, it is imperative to not simply train once on a singular skill, but to create a cumulative training program that build in expertise, customizing prompts and use cases to role types. We found that this training structure saw the best engagement and had the biggest impact. AI is not a one-size-fits-all solution. The more we trained, the better usage results we saw. There was value in repetition. We'll dive deeper into this in our next blog post in this series, where we'll discuss how to create an organization of power users on a given technology.
Measure: Continuous assessment, refinement, and impact
Finally, it's well known the value that measurement brings to project go-to-market. If you don't measure, you can’t truly understand if you’ve solved the problem that you set out to address. This is no different with generative AI solutions, however it is not always obvious how to measure the desired outcome – so be prepared to get a little creative. We took multiple approaches, inclusive of internal surveys and interviews, and looking at aggregated telemetry data, to understand how our organization is transforming. But make no mistake, while the paradigm shift that is artificial intelligence may bring new challenges, it also brings excitement and the ability to tackle things that used to be impossible. Capture that excitement and put it to use for your business.
Bringing it all together
We hope you’re as excited as we are to learn more about how generative AI can revolutionize productivity and unleash the potential of marketers everywhere. Stay tuned for our next blog post and until then, happy creating!
Help us improve Microsoft Advertising
Your input and insights are crucial in shaping and improving our product. You can use the Microsoft Advertising Feedback portal, in-product feedback, X, and as always, contact Support.
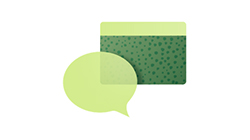
Your input makes us better
Take our quick 3-minute survey and help us transform your website experience.